Home / Spend Control and Financial Forecast with Predictive Analytics
Spend Control and Financial Forecast with Predictive Analytics
- Last updated:
- Blog
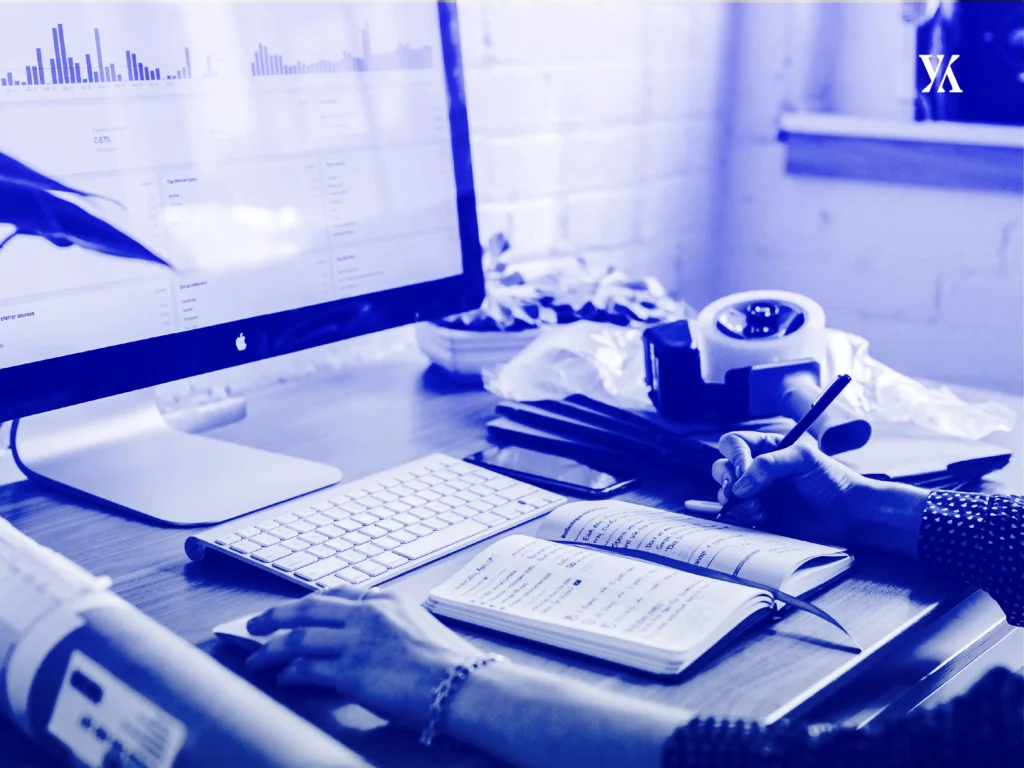
No need for a crystal ball to predict the future: With Artificial Intelligence (AI), you can see tomorrow’s financial trends today and tackle spending surprises before they even hit your budget. Let us explain how forecasting with predictive data analytics works and can help your business!
What is predictive analytics? Definition and importance
Predictive analytics uses historical data, statistical algorithms, and machine learning techniques to forecast future outcomes and trends. It transforms traditional budgeting and forecasting from static, estimation-based processes into dynamic, data-driven exercises. This approach allows companies to generate more accurate financial projections by identifying patterns and correlations within past data. As a result, businesses can better anticipate future financial needs, optimise resource allocation, and reduce the risk of budget overruns. Making informed, proactive decisions based on reliable predictions provides a significant competitive edge in today’s rapidly changing business environment.
Predictive analytics tools improve data quality and integration by consolidating information from data sources into a unified view, ensuring consistency and reliability. It enables continuous monitoring of key metrics, allowing businesses to detect deviations and trends in real-time. This capability validates that forecasts and budgets remain accurate and relevant. Predictive analytics also supports ongoing adjustments by providing insights that help businesses quickly adapt their strategies and resources in response to changing market conditions.
How artificial intelligence helps with predictive analytics
AI can transform predictive analytics by automating complex data analysis, uncovering deeper insights into big data and identifying patterns at high speeds. AI enhances prediction accuracy and adapts dynamically as new data emerges, ensuring forecasts stay relevant amidst changing conditions. It simplifies the implementation of predictive analytics by automating data collection, processing, and model-building, making these advanced capabilities accessible to businesses of all sizes without needing extensive data science teams. Additionally, AI integrates seamlessly with existing systems, providing real-time, actionable insights that empower companies to make confident, data-driven decisions efficiently.
Benefits of predictive analytics
As part of business intelligence, predictive analytics enhances decision-making by forecasting future trends and outcomes. It enables organisations to address potential challenges and seize opportunities proactively.
Revenue growth: Predictive analytics can help businesses grow by enabling them to anticipate market trends, customer behaviours, and demand fluctuations. You can optimise sales strategies, product offerings, and marketing efforts by leveraging these insights.
Improved accuracy: Predictive analytics help predict future outcomes by enhancing the accuracy of forecasts and business models using historical data and advanced algorithms. Errors in financial projections could be minimised, businesses can plan more effectively, and resources can be allocated where they are most needed.
Optimised decision-making: Data-driven insights through predictive analytics empower businesses to make better decisions. The ability to predict potential outcomes allows decision-makers to weigh risks and benefits more accurately.
Budget adjustments: Predictive analytics enables dynamic budgeting by continuously monitoring financial performance and forecasting future needs. This allows companies to make real-time budget adjustments, reallocating funds to areas that require more investment or cutting back where less is needed.
Cost control: By predicting potential cost drivers and identifying inefficiencies, companies can anticipate and mitigate cost overruns, optimise procurement strategies, and avoid unnecessary expenditures with predictive analytics. This proactive approach to cost management ensures that resources are used efficiently, maximising profit margins.
Resource optimisation: Predictive analytics allows businesses to optimise the allocation of resources, such as personnel, inventory, and capital, by forecasting demand and operational needs. This ensures that resources are available where and when they are most needed, reducing waste and improving operational efficiency. Effective resource optimisation leads to better service delivery and higher customer satisfaction.
Fraud prevention: Identifying and preventing fraud by analysing transaction patterns and detecting anomalies is a crucial part of predictive analytics to safeguard your business. By flagging these irregularities in real-time, companies can take immediate action to prevent losses, protect assets, and maintain the integrity of their operations.
Yokoy Expense
Streamline your travel and expense management
Say goodbye to manual data entry, lost receipts, and complicated reimbursements. Yokoy handles everything from start to finish, for simple T&E management at any scale.
How it works: components of predictive analytics
One of the core aspects of predictive analytics is predictive modelling, which involves creating mathematical models that can forecast future financial outcomes based on historical data. Predictive analytics models provide the framework for predicting trends, behaviours, and events.
Each of these predictive models—classification, outliers, and time series—plays a vital role in building accurate, reliable predictive analysis that informs business decisions and helps companies stay ahead of the curve. By integrating these models, businesses can gain a deeper understanding of their data, predict future demands and outcomes with greater precision, and respond proactively to emerging trends and challenges.
Classification model
The classification model categorises data into predefined classes or categories. It is beneficial in scenarios where businesses must make decisions based on groupings, such as credit risk assessment, customer segmentation, seasonality or fraud detection. The model analyses various factors — such as payment history, income, and credit score — to classify the customer as high-risk or low-risk. Classification models help to make decisions that minimise risks and maximise opportunities.
Outliers model
The outliers model focuses on identifying data points that deviate significantly from the norm, which could indicate unusual or unexpected events. In financial forecasting, outliers could represent anomalies such as sudden spikes in expenses for raw materials, unexpected drops in revenue, or irregular financial service transactions. The outliers model is essential for maintaining the accuracy of predictive analytics, as it helps filter out noise and ensures that predictions are based on reliable data.
Time series model
The time series model is used to analyse input or recorded data at specific intervals, allowing businesses to forecast trends and patterns over time. This model is particularly important in financial forecasting, where understanding how variables evolve over time is crucial. Time series models are essential for budgeting and planning, as they provide a temporal context to predictions, helping businesses anticipate changes and adjust their strategies accordingly.
Machine learning algorithms
Machine learning algorithms are another important part of predictive analytics, driving the ability to uncover patterns and predict future outcomes accurately. These algorithms learn from historical data by identifying complex relationships and correlations that might not be immediately apparent through traditional analysis. Common machine learning algorithms used in predictive analytics include regression analysis, decision trees, random forests, and neural networks. These tools can handle large datasets from big data, making sense of diverse and vast information to generate reliable predictions. The algorithms continuously process data over time, refine their accuracy, adapt to new patterns, and improve forecasts.
Integration with business processes
Integrating predictive analytics with business intelligence is crucial for maximising its impact. Seamlessly embedding predictive models into existing workflows drives proactive decision-making across the business: Finance, operations, marketing, and human resources can profit from predictive analytics.
The key to successful integration is aligning predictive analytics with strategic objectives and ensuring that the insights generated directly inform and enhance business operations. By embedding predictive analytics into daily processes, companies can transform raw data into actionable intelligence, leading to improved efficiency, cost savings, and a stronger competitive edge.
Yokoy Expense
Manage expenses effortlessly
Streamline your expense management, simplify expense reporting, and prevent fraud with Yokoy’s AI-driven expense management solution.
Predictive analytics in different business areas
Finance: Predictive analytics transforms finance by enhancing decision-making and performance through accurate revenue, expenses, and cash flow forecasts. It aids in risk management by identifying potential financial risks, supports investment strategies with stock price predictions, and optimises budgets by providing insights into future financial needs and adjusting dynamically.
Inventory management: In inventory management, predictive analytics helps optimise inventory levels by forecasting demand more precisely. For instance, in supply chain management, predictive analytics can forecast seasonality demand, reducing the risk of overstocking and stockouts and helping reduce waste. This improves inventory turnover rates, minimises storage costs, and enhances cash flow.
Supply chain optimisation: Predictive analytics is crucial for supply chain optimisation, as it enhances visibility and efficiency across the entire supply chain. Forecasting tools enable better procurement, production, and distribution planning, leading to improved coordination and reduced lead times. By analysing data from various sources, predictive analytics supports optimising inventory levels, transportation routes, and overall supply chain performance, contributing to cost savings and improved customer satisfaction.
Human Resources (HR): In HR, predictive analytics is used to enhance talent management and workforce planning. It helps in anticipating hiring needs by analysing trends in employee turnover, recruitment data, and business growth projections. Predictive models can identify high-potential candidates, assess employee performance, and predict future training requirements. It aids in improving employee retention by analysing factors contributing to job satisfaction and predicting potential attrition risks.
Operations: Predictive analytics boosts operational efficiency by forecasting maintenance needs, reducing downtime, and extending asset life. It helps anticipate production bottlenecks, optimise scheduling, and improve resource allocation while supporting quality control to prevent defects. This proactive approach enhances productivity, lowers costs, and streamlines business processes.
Predictive analytics made easy with AI-powered expense management solution Yokoy
Yokoy’s expense management software leverages AI to transform financial forecasting and decision-making. Our advanced AI techniques automate data input and processing, extracting key information from receipts, invoices, and transactions with high accuracy. This forms a robust foundation for predictive analytics by ensuring that financial data is consistently accurate and current. Real-time data analysis helps identify spending patterns, trends, and anomalies, which helps forecast future expenses and detect unusual transactions. This enables your business leaders to make smart decisions and adjust their budgets dynamically based on accurate, current data.
Yokoy’s integrated predictive analytics models generate precise estimates for budgeting and financial planning by analysing your historical data and current market trends. Yokoy’s expense management system excels in identifying irregularities in data sets, thereby preventing potential disruptions. Customisable reporting and insights allow businesses to tailor analyses to their specific needs, improving financial visibility and decision-making.
Additionally, Yokoy’s seamless integration with existing financial systems ensures that predictive insights are consistent across all business functions, optimising overall financial performance and resource allocation.
Next steps
If you want to learn more about how Yokoy’s predictive AI analysis can support you with financial forecasting and control spending, book your demo now!
In this article
See intelligent spend management in action
Book a demoRelated content
If you enjoyed this article, you might find the resources below useful.